In recent years, the landscape of AI has shifted dramatically, largely thanks to the rise of open-source initiatives. No longer limited to tech giants with deep pockets, cutting-edge advancements in AI, particularly in the realm of generative models, have become more accessible to developers and organizations worldwide. This democratization of innovation fosters collaboration and rapid growth while raising critical questions about ethics, data security, and inclusivity.
The convergence of open-source software and generative AI has led to a renaissance of creativity, allowing anyone with technical skills to build models that can generate human-like content. This isn’t just a technological transformation but a societal one. As more people gain access to these powerful tools, the way we think about content creation, automation, and even human-computer interactions is changing rapidly. However, the open-source revolution also brings to the fore ethical concerns about how AI is developed and used. Let’s dive into how open-source technology is shaping the future of generative AI and its broader implications for society.
What is Open Source in Generative AI?
Open source refers to software or technology freely available for anyone to use, modify, and distribute. In the context of generative AI, this openness allows developers to build on existing models to create art, music, text, or even software. By leveraging powerful algorithms like GPT-4, DALL·E, or Stable Diffusion, open-source generative AI encourages participation and innovation.
The open-source movement originated in the software community, where developers shared their code to foster collaboration and drive rapid improvements. Now, with generative AI, this ethos has been extended to artificial intelligence. Generative AI models are designed to produce content—whether it’s text, visuals, or other media—based on training data. These models learn from vast amounts of data and can then generate new, creative outputs that mimic human work. The exciting part of open-source generative AI is that anyone, from startups to individuals with minimal resources, can contribute to these models’ development and innovation.
Imagine a landscape where anyone with a computer can develop AI models that produce human-like content, contributing to fields as diverse as entertainment, medicine, or even environmental science. This accessibility is changing how AI is perceived and utilized in daily life.
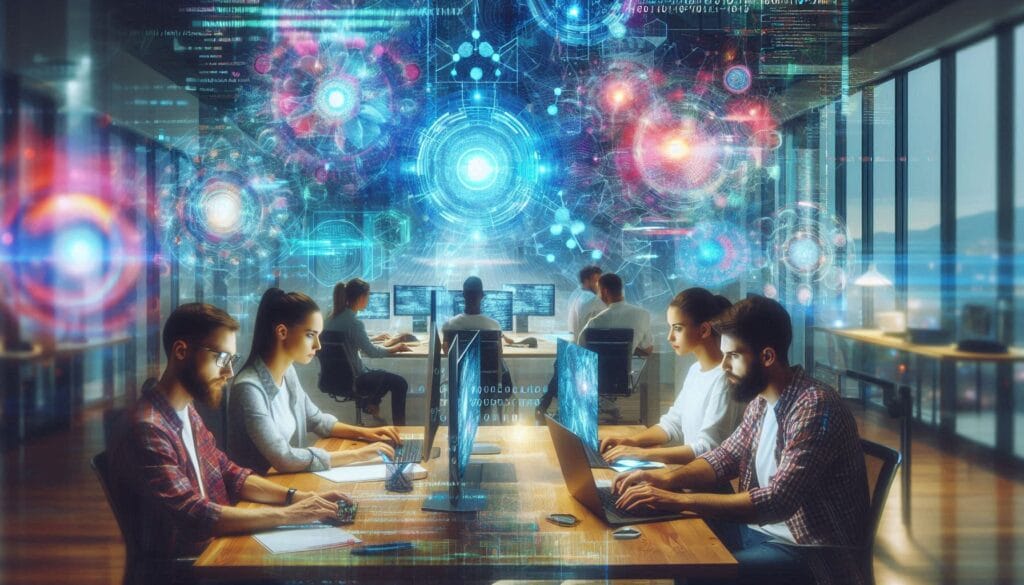
Why is the Shift Important?
The transition towards open-source in generative AI is crucial for several reasons. First, it levels the playing field. Previously, only large corporations with vast resources could lead AI innovation. Today, startups, universities, and independent researchers have access to powerful tools to push the boundaries of what’s possible. Open-source AI enables smaller players to contribute meaningfully to technological advancements without massive financial backing.
Additionally, open source fosters transparency and trust. Unlike proprietary models, which are often black boxes, open-source models allow for peer review, ensuring they are safe, unbiased, and robust. This openness leads to better community-driven scrutiny, resulting in AI systems that are more fair and accountable. Transparency also means that developers can tweak these models to fit their specific needs, encouraging more customized and useful AI applications across various industries.
The shift is also important because it democratizes AI knowledge. Educational institutions can now use open-source AI tools to train the next generation of AI developers, ensuring that knowledge is not limited to a privileged few.
Real-World Examples:
- Hugging Face: Hugging Face provides a platform for developers to access pre-trained AI models, especially for natural language processing tasks. Their open-source models have been adopted by industries ranging from customer service to healthcare, creating more inclusive and dynamic AI applications. By allowing developers to fine-tune models on their own datasets, Hugging Face has empowered a wide range of users to implement AI solutions without the need for in-house model development expertise. Learn more about Hugging Face’s mission and platform here.
- Stable Diffusion: Stable Diffusion is an open-source text-to-image model that has sparked a wave of creativity, from individual artists to large-scale ad agencies. By giving everyone access to cutting-edge AI, it has enabled the creation of stunning visuals with just a text prompt. The accessibility of Stable Diffusion has encouraged innovation in fields such as advertising, where visuals can be generated on demand, saving time and resources. It has also helped individuals in smaller creative sectors, such as indie game development, level up their artistic output. Discover how Stable Diffusion is transforming creative industries here.
- EleutherAI: This grassroots research collective is working to produce large-scale open-source language models that rival those from private companies like OpenAI. Their model GPT-NeoX is being used in applications across industries, from automating customer support to conducting advanced research in linguistics. By providing open access to their models, EleutherAI is helping bridge the gap between small enterprises and the giant AI corporations. Find out more about EleutherAI’s open-source contributions here.
Impact on User Engagement:
Generative AI is fundamentally changing how users interact with technology. From personalized content recommendations to automated creative processes, the ability to engage users in more meaningful and natural ways is driving adoption in sectors like e-commerce, entertainment, and even education. By making AI more intuitive and accessible, open-source tools are revolutionizing how businesses engage with their audiences.
In the world of e-commerce, for example, generative AI can create personalized shopping experiences by generating product recommendations or crafting tailor-made content that resonates with individual users. In education, AI-powered tutoring tools are being developed to engage students with personalized learning materials and immediate feedback, enhancing the learning experience.
Challenges:
While the open-source revolution in generative AI brings numerous benefits, it also introduces significant challenges. One of the major concerns is data privacy. Open models can sometimes be vulnerable to misuse, raising issues around sensitive information leaks. Additionally, the very nature of open source means that bad actors could exploit models for unethical purposes.
Another challenge is bias in AI models. Since many open-source AI models are trained on publicly available datasets, there is a risk that these datasets contain inherent biases, which may be reflected in the models’ outputs. Developers must take care to address these biases through robust training methods and ongoing scrutiny.
For a deep dive into these challenges:
The Dark Side of Open Source AI: Bias and Privacy Risks Read more here.
Future Trends:
Looking ahead, we can expect even more widespread adoption of open-source generative AI as models become more advanced and accessible. Key trends to watch include the integration of multimodal AI, where models can understand and generate across different media like text, images, and sound. This will allow developers to build even more complex and intelligent systems capable of interacting with users in new and engaging ways.
Additionally, AI ethics will continue to be a critical conversation, as more stakeholders push for responsible AI development. Developers and organizations will need to work together to ensure that these open-source models are used for the greater good, avoiding pitfalls such as deepfake abuse or discriminatory practices.
Final Thoughts:
The rise of open-source generative AI represents a seismic shift in the world of technology and innovation. It has opened the doors for creators, developers, and businesses to experiment and contribute to cutting-edge developments. While it democratizes AI, it also challenges us to think deeply about the ethical implications of such power. As we continue to explore the possibilities, the balance between innovation and responsibility will define the next chapter in AI’s evolution.
- “Unlocking the power of Multimodal AI.” Read here.