In today’s data-driven world, data engineering forms the backbone of artificial intelligence (AI) projects. With the growing volume, variety, and velocity of data, building efficient and scalable data pipelines has become essential. AI models thrive on high-quality data, and the processes that transform raw data into usable formats can either make or break AI initiatives. In this blog, we’ll explore the core concepts of data engineering, how it plays a critical role in AI projects, and the real-world applications that are driving innovation in this field.
What is Data Engineering?
Data engineering involves designing, building, and maintaining systems that handle large volumes of data. It focuses on creating robust data pipelines that can process data from various sources, ensuring it’s clean, organised, and ready for analysis or modelling. These pipelines are crucial in AI projects because they fuel machine learning algorithms with the right data at the right time.
Why is the Shift to Advanced Data Engineering Important?
As AI projects scale, the need for efficient data pipelines has increased. Moving from simple, manual data handling to advanced, automated pipelines ensures that AI systems can process vast amounts of data in real-time, leading to more accurate and timely insights.
Real-World Examples
Netflix’s Personalized Recommendations
Netflix uses data pipelines to process user data and deliver personalised recommendations based on viewing history. These pipelines ensure that the system can scale to millions of users while providing real-time suggestions.
How Netflix Uses Data Engineering
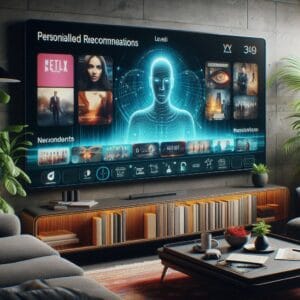
Uber’s Real-Time Data Processing
Uber’s data pipelines process real-time data from millions of trips to optimise routes, reduce wait times, and provide dynamic pricing.
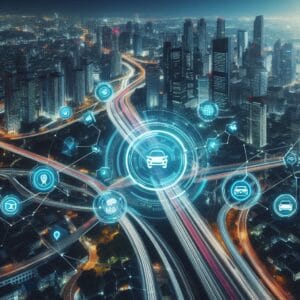
- Facebook’s Ad Targeting
Facebook uses robust data pipelines to analyze user behaviors and interactions. These pipelines allow the platform to serve highly targeted advertisements by processing and organizing massive datasets in real-time, ensuring ads are personalized and relevant to each user’s preferences and online activity.
How Facebook Optimises Ad Targeting Using Data Pipelines
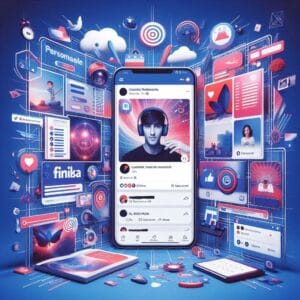
Impact on User Engagement
The role of data pipelines in AI has drastically improved how businesses interact with users. Whether it’s providing personalized recommendations, real-time information, or better decision-making tools, data pipelines enhance user engagement by offering more intuitive, relevant, and timely experiences.
Challenges
Despite the immense value of data pipelines, building them comes with challenges. Data privacy concerns, biases in AI models, and the need for high-quality, labeled data are some of the key issues that must be addressed.
Future Trends
Looking ahead, data pipelines will only become more crucial as AI systems evolve. Key trends include the integration of real-time streaming data, AI-driven automation of data management tasks, and the adoption of multi-cloud and hybrid-cloud architectures to scale data engineering capabilities.
Final Thoughts
Data engineering is an indispensable part of AI projects, providing the foundation for machine learning and analytics. As we’ve explored, building robust data pipelines ensures that AI models have access to accurate, timely data, which leads to more meaningful insights. As AI continues to grow, so will the demand for efficient data pipelines.